Chris will present his M.Sc. thesis “Investigating the capability of UAV imagery in AI-assisted mapping of Refugee Camps in East Africa” on Friday 16th of September at 2pm. From his abstract: ” Refugee camps and informal settlements provide accommodation to some of the most vulnerable populations, with many of them located in Sub-Saharan Africa. Many of
these settlements lack up-to-date geoinformation that we take for granted in developed settlements. Having up-to-date maps on their dimension, spatial layout is important. They are essnetial tools for assisting administration tasks such as crisis intervention, infrastructure development, and population estimates which encourage economic productivity. In the OSM ecosystem, there is a disparity between built-up being digitised in the developed and the developing areas. This data enequality are results of multiple reasons ranging from a lack of commercial interest to knowledge gaps in data contributors. Such disparity can be reduced using assisted mapping technology. Very High Resolution satellite imagery and Machine Learning based methods can exploit the textural, spectral, and morphological characteristics of VHR imagery are commonly used to extract geospatial and imagery of these complex environments. Recent advances in Deep Learning based Computer Vision, however, might be able to address these issues. This study is connected to a larger initiative to open-source the AI assisted mapping platform in the current Humanitarian OpenStreetMap Team’s ecosystem.
To investigated the capabilities of applying Deep Learning semantic segmentation methods for delineating building footprints in refugee camps from open-data drone imagery. The objective of this study is to test the U-Net and several variations of the
architectures’ performance for building footprint mapping, The performance of the different Deep Learning models on datasets of different complexity were collected. A comparison of the models responses using class-based accuracy assessments metrics allows evaluation into which level of data quality is required to achieve acceptable classification results when scaling Deep Learning assisted mapping efforts in the context of humanitarian mapping.
This study demonstrats the ability to use Deep Learning semantic segmentation to perform building segmentation in complex humanitarian applications. Having increased access to open-data Very High Resolution drone imagery such as the OpenAerialMap initiative is an advantage to building AI-assisted humanitarian mapping. The study evaluated various U-Net based architectures and data input setup. Yet, the variation of the results not only emphasised the complexity of Deep Learning based methods, but also indicate that further efforts will be needed to focus on the selection of suitable network architectures as they show varying resilience towards imperfect reference samples.
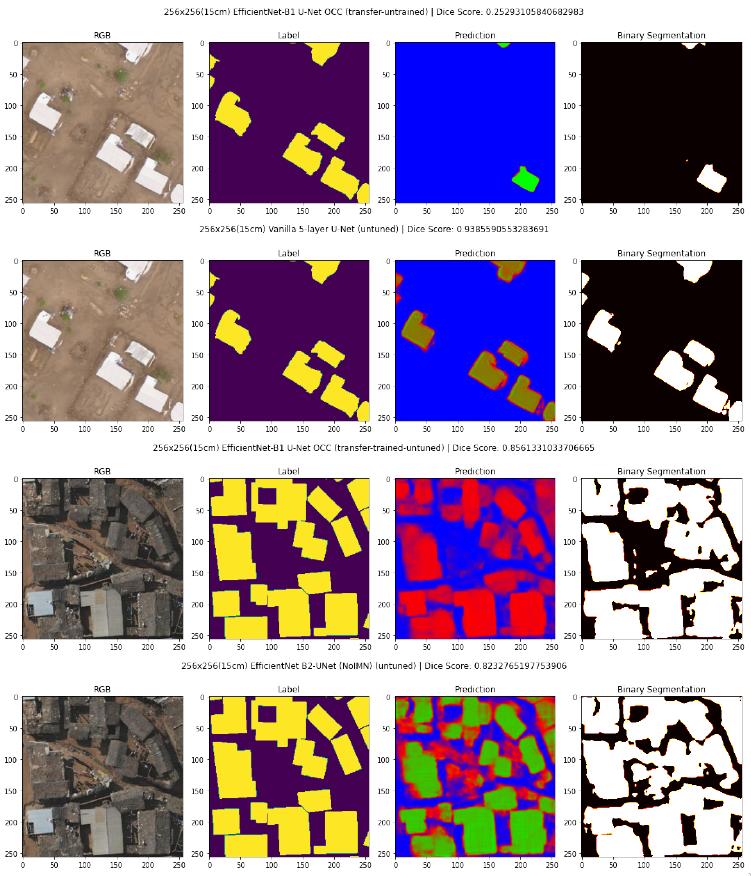
supervisors: Hannes Taubenböck (DFD-DLR), Martin Wegmann, Matthias Weigand (DFD-DLR), Emran Alchikh Alnajar (Humanitarian OpenStreetMap)