About
Christian is with the team “Modeling and geostatistical analysis” at the German Aerospace Center (DLR). He worked on several topics related to renewable energy, earthquake risk assessment, and methods for remote sensing data processing. His current research interests include techniques of multimodal remote sensing, machine learning, and pattern recognition, specifically applied to the field of natural hazard risk research.
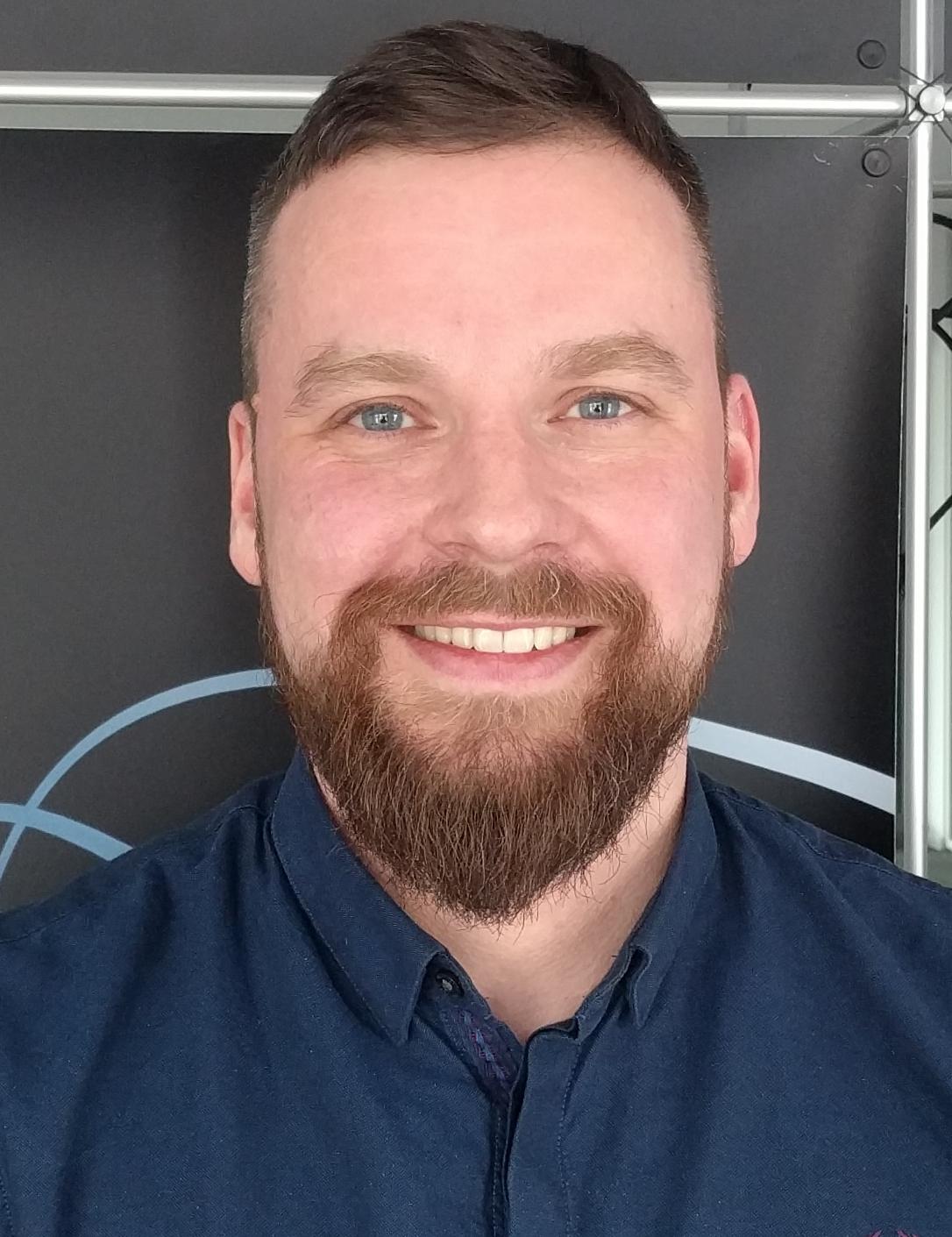
Dr. Christian Geiss, DLR-EOC
Recent News
MSc: predicting forest understory canopy cover
The M.Sc thesis by Bastian Schumann focused on a LiDAR-based approach to combine structural metrics and forest habitat information for causal and predictive models of under-story canopy cover. The data base used consisted of a bi-temporal LiDAR dataset as well as two...
Explore species-environment interaction
Analyzing species-environment interaction is feasible using various data and method. An increasing technology is the tracking of animals and especially its linkage to remote sensing, as covered in AniMove.org. However, with this technology new challenges have to be...
MSc by Asja Bernd: “Mind the Gap: A Global Analysis of Grassland Fragmentation using MODIS Land Cover Data”
The MSc thesis by Asja Bernd titled “Mind the Gap: A Global Analysis of Grassland Fragmentation using MODIS Land Cover Data” is handed in. Very interesting results on global grassland fragmentation. Read the abstract:Around the world, grassland and savannah...
MSc on deforestation in Myanmar and its drivers
The MSc by Andrea Hess “Deforestation in Myanmar – what can we say about causes?” has been handed in. It has been supervised by Peter Leimgruber (Smithsonian Conservation Biology Institute) and Martin Wegmann. Deforestation in the tropics is a global issue. Tropical...
Deployment of a multi-classifier approach to improve land cover classification accuracy
This study will examine whether the application of hybrid classifiers increases the classification accuracy in comparison to a single classifier. A combination between parametric and non-parametric classifiers will be applied and their performance will be assessed....
Stereo photogrammetry for multi-temporal surface models based on aerial imagery
The data provided by aerial imagery are amongst the oldest sources of spatially explicit information for modern-time environmental management. These data are often captured over landscape-level domains using overlapping flight stripes to enable stereo photogrammetric...
Estimation of actual evapotranspiration in irrigation agricultural area of Uzbekistan using high resolution multi-frequent synthetic remote sensing data
Actual evapotranspiration (ETact) is an essential component of the water balance and its determination for large areas is difficult on regional scale and can be explored within an innovation laboratory. The use of remote sensing data to determine ETact is particularly...
Object based sequential masking classification using SAR and optical data
Remote sensing based crop mapping is still challenging when just relying on optical information as the only data source. Due to the unavailability of adequate optical satellite images the integration of SAR is promising and can be explored within an innovation...
Radiometric calibration, speckle reduction, geometric and terrain correction are essential preprocessing steps for SAR data. Within an innovation laboratory you will explore the different calibration and data preparation techniques for ground detected and single look...
Surface model creation from multi-source remote sensing data
Nowadays there are plenty of 3D data sources which offer the possibility to model and monitor structural attributes of the landscape via the derivation of terrain, surface and canopy models. The core idea of this research project within an innovation laboratory is to...